We need to talk about how good AI is getting
New York Times
last updated: Aug 28,2022
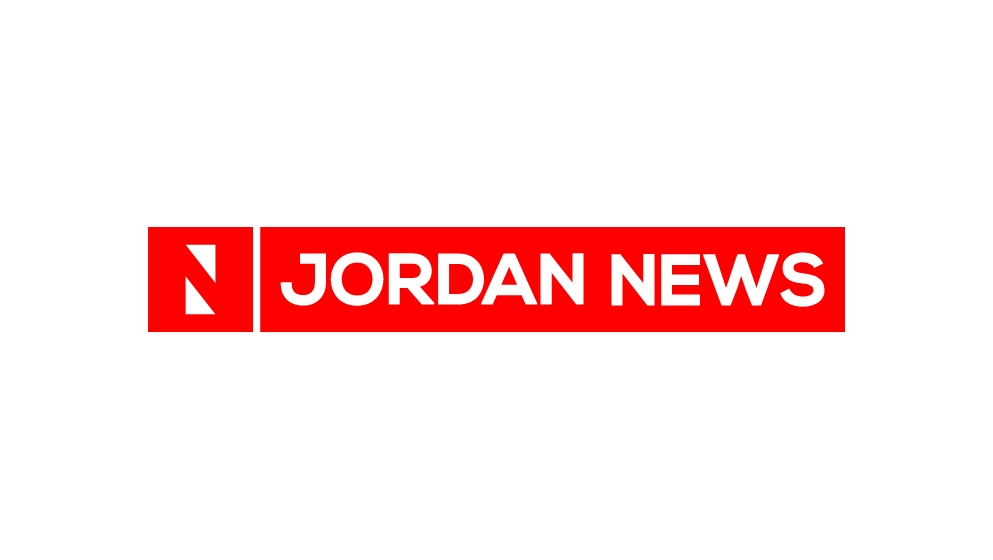
For the past few days, I have been playing around with
DALL-E 2, an app developed by the San Francisco company OpenAI that turns text
descriptions into hyper-realistic images.اضافة اعلان
OpenAI invited me to test DALL-E 2 (the name is a play on Pixar’s WALL-E and artist Salvador Dalí) during its beta period, and I quickly got obsessed. I spent hours thinking up weird, funny, and abstract prompts to feed the AI — “a 3D rendering of a suburban home shaped like a croissant”, “an 1850s daguerreotype portrait of Kermit the Frog”, “a charcoal sketch of two penguins drinking wine in a Parisian bistro.” Within seconds, DALL-E 2 would spit out a handful of images depicting my request — often with jaw-dropping realism.
What is impressive about DALL-E 2 is not just the art it generates. It is how it generates art. These are not composites made out of existing internet images — they’re wholly new creations made through a complex AI process known as “diffusion,” which starts with a random series of pixels and refines it repeatedly until it matches a given text description. And it is improving quickly — DALL-E 2’s images are four times as detailed as the images generated by the original DALL-E, which was introduced only last year.
DALL-E 2 got a lot of attention when it was announced this year, and rightfully so. It is an impressive piece of technology with big implications for anyone who makes a living working with images — illustrators, graphic designers, photographers, and so on. It also raises important questions about what all of this AI-generated art will be used for, and whether we need to worry about a surge in synthetic propaganda, hyper-realistic deepfakes, or even nonconsensual pornography.
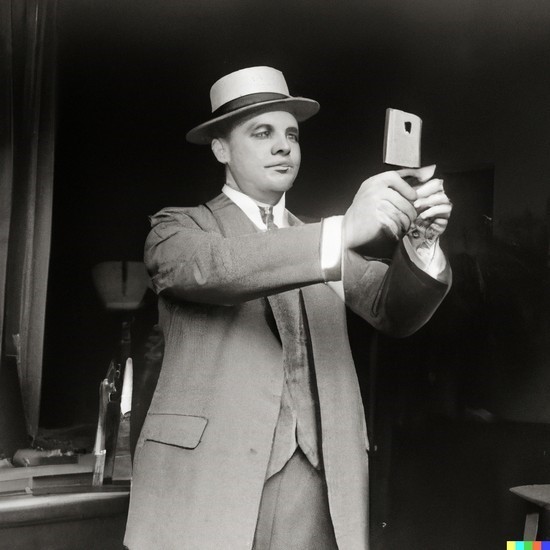
“Black-and-white vintage photograph of a 1920s mobster taking a selfie,” an image generated using OpenAI’s DALL-E 2 artificial intelligence program.
But art is not the only area where artificial intelligence has been making major strides.
Over the past 10 years — a period some AI researchers have begun referring to as a “golden decade” — there’s been a wave of progress in many areas of AI research, fueled by the rise of techniques like deep learning and the advent of specialized hardware for running huge, computationally intensive AI models.
Some of that progress has been slow and steady — bigger models with more data and processing power behind them yielding slightly better results.
But other times, it feels more like the flick of a switch — impossible acts of magic suddenly becoming possible.
Just five years ago, for example, the biggest story in the AI world was AlphaGo, a deep learning model built by Google’s DeepMind that could beat the best humans in the world at the board game Go. Training an AI to win Go tournaments was a fun party trick, but it was not exactly the kind of progress most people care about.
But last year, DeepMind’s AlphaFold — an AI system descended from the Go-playing one — did something truly profound. Using a deep neural network trained to predict the three-dimensional structures of proteins from their one-dimensional amino acid sequences, it essentially solved what’s known as the “protein-folding problem,” which had vexed molecular biologists for decades.
This summer, DeepMind announced that AlphaFold had made predictions for nearly all of the 200 million proteins known to exist — producing a treasure trove of data that will help medical researchers develop new drugs and vaccines for years to come. Last year, the journal Science recognized AlphaFold’s importance, naming it the biggest scientific breakthrough of the year.
Or look at what is happening with AI-generated text.
Only a few years ago, AI chatbots struggled even with rudimentary conversations — to say nothing of more difficult language-based tasks.
But now, large language models like OpenAI’s GPT-3 are being used to write screenplays, compose marketing emails and develop video games. (I even used GPT-3 to write a book review for this paper last year — and, had I not clued in my editors beforehand, I doubt they would have suspected anything.)
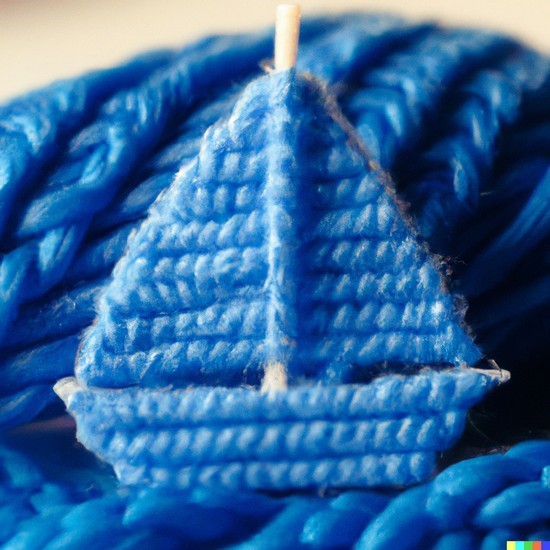
“A sailboat knitted out of blue yarn,” an image generated using OpenAI’s DALL-E 2 artificial intelligence program.
AI is writing code, too — more than 1 million people have signed up to use GitHub’s Copilot, a tool released last year that helps programmers work faster by automatically finishing their code snippets.
Then there’s Google’s LaMDA, an AI model that made headlines a couple of months ago when Blake Lemoine, a senior Google engineer, was fired after claiming that it had become sentient.
Google disputed Lemoine’s claims, and lots of AI researchers have quibbled with his conclusions. But take out the sentience part, and a weaker version of his argument — that LaMDA and other state-of-the-art language models are becoming eerily good at having humanlike text conversations — would not have raised nearly as many eyebrows.
In fact, many experts will tell you that AI is getting better at lots of things these days — even in areas, such as language and reasoning, where it once seemed that humans had the upper hand.
There is still plenty of bad, broken AI out there, from racist chatbots to faulty automated driving systems that result in crashes and injury. And even when AI improves quickly, it often takes a while to filter down into products and services that people actually use. An AI breakthrough at Google or OpenAI today doesn’t mean that your Roomba will be able to write novels tomorrow.
But the best AI systems are now so capable — and improving at such fast rates — that the conversation in Silicon Valley is starting to shift. Fewer experts are confidently predicting that we have years or even decades to prepare for a wave of world-changing AI; many now believe that major changes are right around the corner, for better or worse.
There are, to be fair, plenty of skeptics who say claims of AI progress are overblown. They will tell you that AI is still nowhere close to becoming sentient, or replacing humans in a wide variety of jobs. They’ll say that models like GPT-3 and LaMDA are just glorified parrots, blindly regurgitating their training data, and that we’re still decades away from creating true AGI — artificial general intelligence — that is capable of “thinking” for itself.
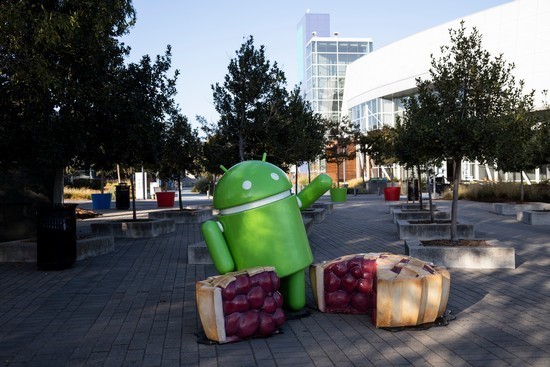
An Android statue welcomes visitors outside the headquarters of Google and its parent company Alphabet Inc., in Mountain View, California, on October 20, 2020.
There are also tech optimists who believe that AI progress is accelerating, and who want it to accelerate faster. Speeding AI’s rate of improvement, they believe, will give us new tools to cure diseases, colonize space, and avert ecological disaster.
I am not asking you to take a side in this debate. All I’m saying is: You should be paying closer attention to the real, tangible developments that are fueling it.
It is a cliché, in the AI world, to say things like “we need to have a societal conversation about AI risk.” There are already plenty of Davos panels, TED talks, think tanks, and AI ethics committees out there, sketching out contingency plans for a dystopian future.
What is missing is a shared, value-neutral way of talking about what today’s AI systems are actually capable of doing, and what specific risks and opportunities those capabilities present.
I think three things could help here.
First, regulators and politicians need to get up to speed.
Because of how new many of these AI systems are, few public officials have any firsthand experience with tools like GPT-3 or DALL-E 2, nor do they grasp how quickly progress is happening at the AI frontier.
Otherwise, we could end up with a repeat of what happened with social media companies after the 2016 election — a collision of Silicon Valley power and Washington ignorance, which resulted in nothing but gridlock and testy hearings.
Second, big tech companies investing billions in AI development — the Googles, Metas, and OpenAIs of the world — need to do a better job of explaining what they are working on, without sugarcoating or soft-pedaling the risks. Right now, many of the biggest AI models are developed behind closed doors, using private data sets, and tested only by internal teams. When information about them is made public, it’s often either watered down by corporate PR or buried in inscrutable scientific papers.
Downplaying AI risks to avoid backlash may be a smart short-term strategy, but tech companies will not survive long term if they’re seen as having a hidden AI agenda that’s at odds with the public interest. And if these companies won’t open up voluntarily, AI engineers should go around their bosses and talk directly to policymakers and journalists themselves.
Third, the news media needs to do a better job of explaining AI progress to non-experts. Too often, journalists — and I admit I’ve been a guilty party here — rely on outdated sci-fi shorthand to translate what’s happening in AI to a general audience. We sometimes compare large language models to Skynet and HAL 9000, and flatten promising machine learning breakthroughs to panicky “the robots are coming!” headlines we think will resonate with readers. Occasionally, we betray our ignorance by illustrating articles about software-based AI models with photos of hardware-based factory robots — an error that is as inexplicable as slapping a photo of a BMW on a story about bicycles.
In a broad sense, most people think about AI narrowly as it relates to us — Will it take my job? Is it better or worse than me at Skill X or Task Y? — rather than trying to understand all of the ways AI is evolving, and what that might mean for our future.
I will do my part, by writing about AI in all its complexity and weirdness without resorting to hyperbole or Hollywood tropes. But we all need to start adjusting our mental models to make space for the new, incredible machines in our midst.
Read more Technology
Jordan News
OpenAI invited me to test DALL-E 2 (the name is a play on Pixar’s WALL-E and artist Salvador Dalí) during its beta period, and I quickly got obsessed. I spent hours thinking up weird, funny, and abstract prompts to feed the AI — “a 3D rendering of a suburban home shaped like a croissant”, “an 1850s daguerreotype portrait of Kermit the Frog”, “a charcoal sketch of two penguins drinking wine in a Parisian bistro.” Within seconds, DALL-E 2 would spit out a handful of images depicting my request — often with jaw-dropping realism.
What is impressive about DALL-E 2 is not just the art it generates. It is how it generates art. These are not composites made out of existing internet images — they’re wholly new creations made through a complex AI process known as “diffusion,” which starts with a random series of pixels and refines it repeatedly until it matches a given text description. And it is improving quickly — DALL-E 2’s images are four times as detailed as the images generated by the original DALL-E, which was introduced only last year.
DALL-E 2 got a lot of attention when it was announced this year, and rightfully so. It is an impressive piece of technology with big implications for anyone who makes a living working with images — illustrators, graphic designers, photographers, and so on. It also raises important questions about what all of this AI-generated art will be used for, and whether we need to worry about a surge in synthetic propaganda, hyper-realistic deepfakes, or even nonconsensual pornography.
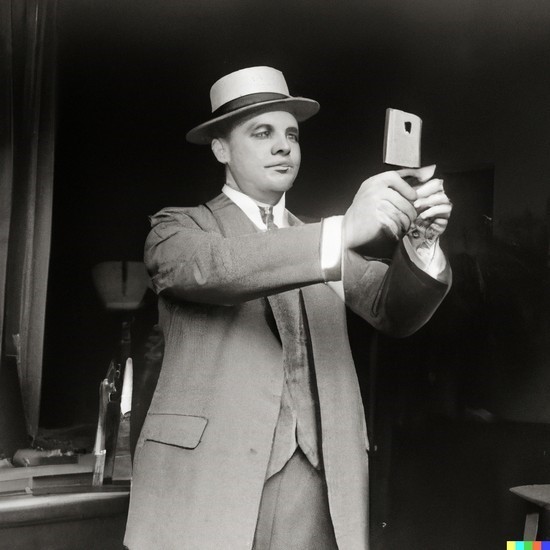
“Black-and-white vintage photograph of a 1920s mobster taking a selfie,” an image generated using OpenAI’s DALL-E 2 artificial intelligence program.
But art is not the only area where artificial intelligence has been making major strides.
Over the past 10 years — a period some AI researchers have begun referring to as a “golden decade” — there’s been a wave of progress in many areas of AI research, fueled by the rise of techniques like deep learning and the advent of specialized hardware for running huge, computationally intensive AI models.
Some of that progress has been slow and steady — bigger models with more data and processing power behind them yielding slightly better results.
But other times, it feels more like the flick of a switch — impossible acts of magic suddenly becoming possible.
Just five years ago, for example, the biggest story in the AI world was AlphaGo, a deep learning model built by Google’s DeepMind that could beat the best humans in the world at the board game Go. Training an AI to win Go tournaments was a fun party trick, but it was not exactly the kind of progress most people care about.
But last year, DeepMind’s AlphaFold — an AI system descended from the Go-playing one — did something truly profound. Using a deep neural network trained to predict the three-dimensional structures of proteins from their one-dimensional amino acid sequences, it essentially solved what’s known as the “protein-folding problem,” which had vexed molecular biologists for decades.
This summer, DeepMind announced that AlphaFold had made predictions for nearly all of the 200 million proteins known to exist — producing a treasure trove of data that will help medical researchers develop new drugs and vaccines for years to come. Last year, the journal Science recognized AlphaFold’s importance, naming it the biggest scientific breakthrough of the year.
Or look at what is happening with AI-generated text.
Only a few years ago, AI chatbots struggled even with rudimentary conversations — to say nothing of more difficult language-based tasks.
But now, large language models like OpenAI’s GPT-3 are being used to write screenplays, compose marketing emails and develop video games. (I even used GPT-3 to write a book review for this paper last year — and, had I not clued in my editors beforehand, I doubt they would have suspected anything.)
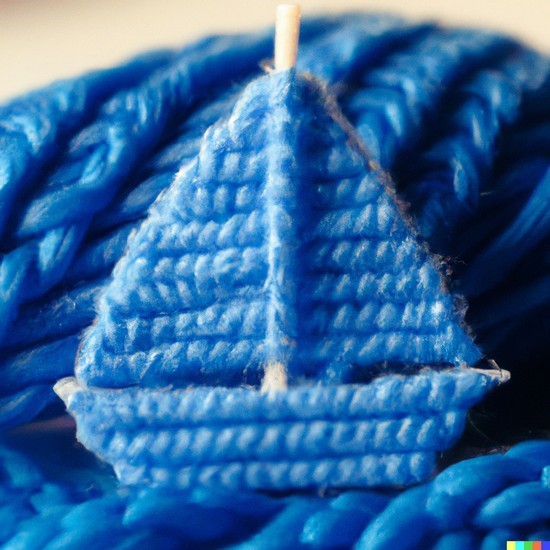
“A sailboat knitted out of blue yarn,” an image generated using OpenAI’s DALL-E 2 artificial intelligence program.
AI is writing code, too — more than 1 million people have signed up to use GitHub’s Copilot, a tool released last year that helps programmers work faster by automatically finishing their code snippets.
Then there’s Google’s LaMDA, an AI model that made headlines a couple of months ago when Blake Lemoine, a senior Google engineer, was fired after claiming that it had become sentient.
Google disputed Lemoine’s claims, and lots of AI researchers have quibbled with his conclusions. But take out the sentience part, and a weaker version of his argument — that LaMDA and other state-of-the-art language models are becoming eerily good at having humanlike text conversations — would not have raised nearly as many eyebrows.
In fact, many experts will tell you that AI is getting better at lots of things these days — even in areas, such as language and reasoning, where it once seemed that humans had the upper hand.
There is still plenty of bad, broken AI out there, from racist chatbots to faulty automated driving systems that result in crashes and injury. And even when AI improves quickly, it often takes a while to filter down into products and services that people actually use. An AI breakthrough at Google or OpenAI today doesn’t mean that your Roomba will be able to write novels tomorrow.
But the best AI systems are now so capable — and improving at such fast rates — that the conversation in Silicon Valley is starting to shift. Fewer experts are confidently predicting that we have years or even decades to prepare for a wave of world-changing AI; many now believe that major changes are right around the corner, for better or worse.
There are, to be fair, plenty of skeptics who say claims of AI progress are overblown. They will tell you that AI is still nowhere close to becoming sentient, or replacing humans in a wide variety of jobs. They’ll say that models like GPT-3 and LaMDA are just glorified parrots, blindly regurgitating their training data, and that we’re still decades away from creating true AGI — artificial general intelligence — that is capable of “thinking” for itself.
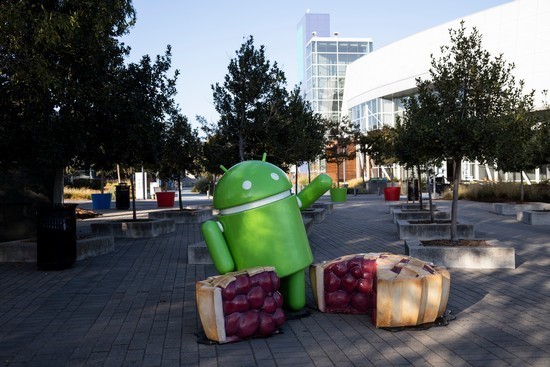
An Android statue welcomes visitors outside the headquarters of Google and its parent company Alphabet Inc., in Mountain View, California, on October 20, 2020.
There are also tech optimists who believe that AI progress is accelerating, and who want it to accelerate faster. Speeding AI’s rate of improvement, they believe, will give us new tools to cure diseases, colonize space, and avert ecological disaster.
I am not asking you to take a side in this debate. All I’m saying is: You should be paying closer attention to the real, tangible developments that are fueling it.
It is a cliché, in the AI world, to say things like “we need to have a societal conversation about AI risk.” There are already plenty of Davos panels, TED talks, think tanks, and AI ethics committees out there, sketching out contingency plans for a dystopian future.
What is missing is a shared, value-neutral way of talking about what today’s AI systems are actually capable of doing, and what specific risks and opportunities those capabilities present.
I think three things could help here.
First, regulators and politicians need to get up to speed.
Because of how new many of these AI systems are, few public officials have any firsthand experience with tools like GPT-3 or DALL-E 2, nor do they grasp how quickly progress is happening at the AI frontier.
AI is writing code, too — more than 1 million people have signed up to use GitHub’s Copilot, a tool released last year that helps programmers work faster by automatically finishing their code snippets.We’ve seen a few efforts to close the gap — Stanford’s Institute for Human-Centered Artificial Intelligence recently held a three-day “AI boot camp” for congressional staff members, for example — but we need more politicians and regulators to take an interest in the technology. (And I do not mean that they need to start stoking fears of an AI apocalypse, Andrew Yang-style. Even reading a book like Brian Christian’s “The Alignment Problem” or understanding a few basic details about how a model like GPT-3 works would represent enormous progress.)
Otherwise, we could end up with a repeat of what happened with social media companies after the 2016 election — a collision of Silicon Valley power and Washington ignorance, which resulted in nothing but gridlock and testy hearings.
Second, big tech companies investing billions in AI development — the Googles, Metas, and OpenAIs of the world — need to do a better job of explaining what they are working on, without sugarcoating or soft-pedaling the risks. Right now, many of the biggest AI models are developed behind closed doors, using private data sets, and tested only by internal teams. When information about them is made public, it’s often either watered down by corporate PR or buried in inscrutable scientific papers.
Downplaying AI risks to avoid backlash may be a smart short-term strategy, but tech companies will not survive long term if they’re seen as having a hidden AI agenda that’s at odds with the public interest. And if these companies won’t open up voluntarily, AI engineers should go around their bosses and talk directly to policymakers and journalists themselves.
Third, the news media needs to do a better job of explaining AI progress to non-experts. Too often, journalists — and I admit I’ve been a guilty party here — rely on outdated sci-fi shorthand to translate what’s happening in AI to a general audience. We sometimes compare large language models to Skynet and HAL 9000, and flatten promising machine learning breakthroughs to panicky “the robots are coming!” headlines we think will resonate with readers. Occasionally, we betray our ignorance by illustrating articles about software-based AI models with photos of hardware-based factory robots — an error that is as inexplicable as slapping a photo of a BMW on a story about bicycles.
In a broad sense, most people think about AI narrowly as it relates to us — Will it take my job? Is it better or worse than me at Skill X or Task Y? — rather than trying to understand all of the ways AI is evolving, and what that might mean for our future.
I will do my part, by writing about AI in all its complexity and weirdness without resorting to hyperbole or Hollywood tropes. But we all need to start adjusting our mental models to make space for the new, incredible machines in our midst.
Read more Technology
Jordan News